What is synthetic data and how does it work?
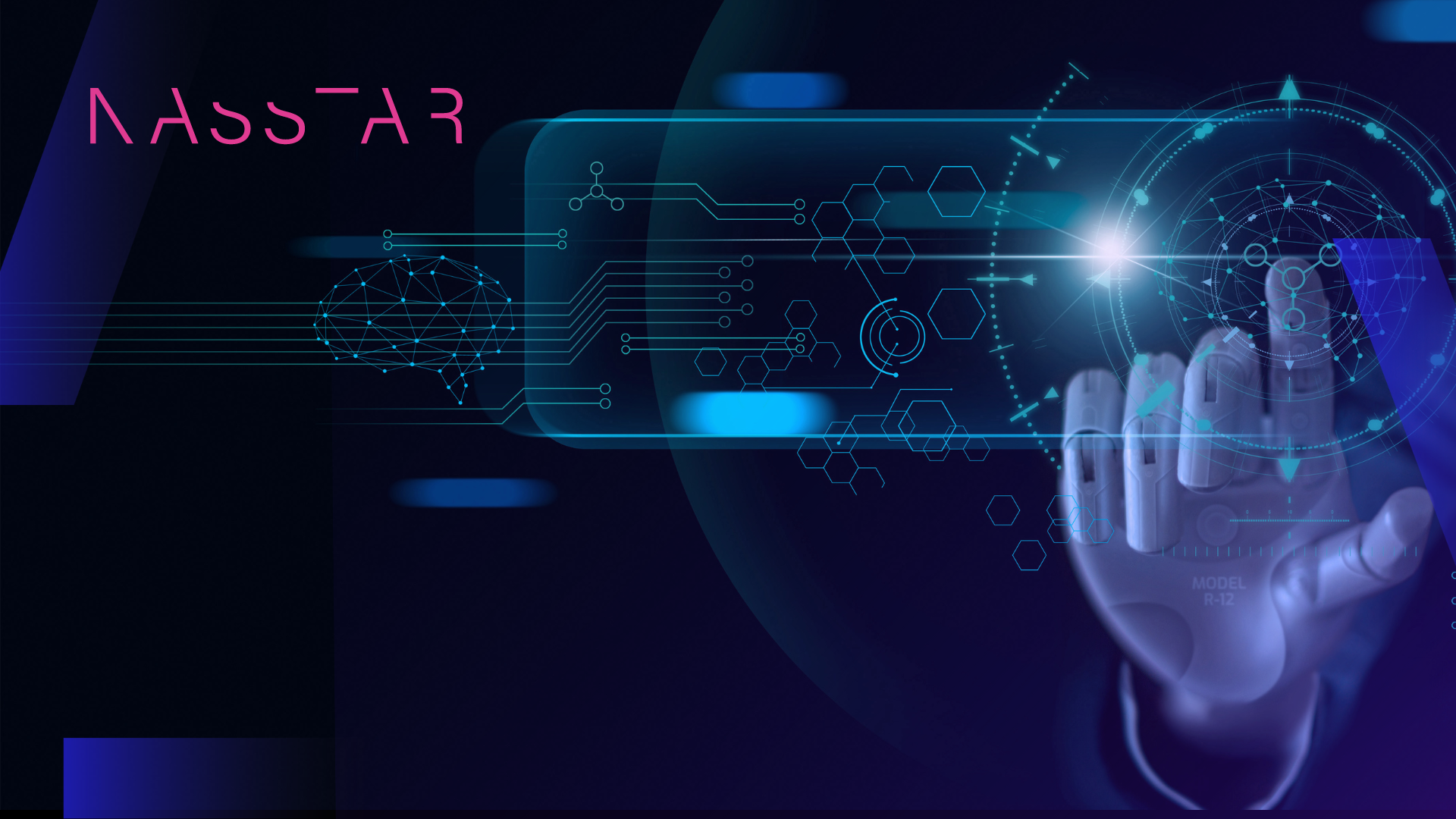
This blog has been expertly reviewed by Andrea Rosales, Data Scientist at Colibri Digital.
Artificial intelligence (AI) and machine learning (ML) are changing the world. Every day, companies are using their data to learn more about customers, improve workflows, and create new products. As a result, two-thirds of businesses increased or maintained AI spending in 2023.
But these deep-learning models need vast amounts of quality data to do their best work.
"Collecting, cleaning, and creating training datasets can be time-consuming, resource-intensive, and expensive. That’s not to mention the potential accessibility, security, and privacy challenges." says Andrea.
Enter synthetic data. Unlike traditional data, synthetic data doesn't copy real-world events or people. It's an artificially generated product — created by algorithms — designed to enhance real-world data. As such, it’s an excellent option for training machine learning models and can be deployed in many other business use cases.
It's anticipated that 60% of data used in learning systems could soon be synthetic. That’s because the advantages of synthetic data cover many bases. Using it, businesses can:
- Address the concerns surrounding privacy regulations or sensitive data usage
- Design data to suit specific business needs
- Test new and hypothetical situations
- Create vast training datasets without the laborious, error-prone task of manual data science
- Save money and time in their data-gathering process.
In this blog, we’ll look at synthetic data, how it helps companies, and who should use it.
What are synthetic datasets?
Fundamentally, synthetic datasets are replicas of real-world data. At the heart of artificial data generation is an algorithm that’s been trained on samples of actual, real-life information. The training process involves the algorithm learning the patterns, correlations, and nuances in the sample data.
Then, the AI model can generate new data – synthetic data – which is statistically similar or close to the original. This makes synthetic data a perfect stand-in for the original dataset. It offers a new dimension to data analysis without compromising on the depth and quality of insights, helping companies create new and meaningful data quickly.
The recent explosion of generative AI has made synthetic data even more accessible. Generative AI models learn from the distribution of training data, allowing them to produce new data that adheres to known patterns.
What are the types of synthetic data?
Nowadays, it’s possible to create various kinds of synthetic data. Each can then be tailored for specific models or applications. For instance, a business might use:
- Unstructured synthetic data like images and videos for training algorithms in object detection and recognition. An example could be an autonomous vehicle company using its synthetic data vault to train self-driving cars.
- Structured synthetic data like tabular data, financial records, or patient information. This use of synthetic data can help highly regulated industries test data and build predictive models without using actual, sensitive customer data.
- Synthetic text can be generated for natural language processing. This type of synthetic model training is especially useful when real text is limited, sensitive, or unavailable. It can also help improve the performance of generative models.
These sophisticated algorithms begin by learning from data samples. They absorb correlations, statistical properties, data structures, and patterns in the original data. Then, once trained, they can produce new data points that are statistically and structurally identical to the original — yet entirely synthetic.
What are the advantages of synthetic data?
Synthetic data generation is a complex process powered by deep generative algorithms. However, it is still often more accessible, faster, and cheaper than collecting real datasets. Some of its key benefits include:
- Synthetic data can bridge the gap between getting real quality data in large volumes by supplementing real-world data and expanding the scope for future projects at scale.
- Synthetic data offers privacy preservation with consistent format and labelling, free from inaccuracies and duplications. This contrasts with real-world data collection, which brings issues like personal data protection, error filtering, labelling, and formatting.
- Managing and analysing synthetic data is generally less costly than data gathering, coupled with greater control over data quality and format.
- With a controlled environment for experimentation and testing, data scientists and machine learning engineers can evaluate the performance of models under different conditions without risking real data.
- Synthetic data can be customised to meet specific requirements, such as mimicking certain statistical distributions, preserving correlations, or introducing specific patterns or anomalies for greater flexibility.
- Training models on more data can positively impact almost any project. Machine learning algorithms benefit from the superior quality of synthetic datasets, while companies see faster development workflows.
- Particularly in sensitive sectors like healthcare or finance, synthetic data offers a secure alternative by mitigating privacy concerns.
With so many potential benefits, it’s easy to see why synthetic data is booming in data science. But are there any challenges?
What are the challenges of synthetic data?
Andrea Rosales, Data Scientist at Colibri Digital, said: “Synthetic data, while revolutionary, is not without its difficulties. Much like real-world information, synthetic data’s effectiveness hinges on the quality of its training set. If this source data is false or unrepresentative, the synthetic data produced will reproduce these issues rather than resolve them.
“Another key concern is bias. Using synthetic data generated by a biased or overfitted model is ineffective and potentially counterproductive. As the old saying goes — garbage in, garbage out.”
To ensure accuracy, integrity and reliability, synthetic data training must build on diverse, well-balanced, real-world data. This will mitigate the risk of magnifying biases and support the development of robust and fair data. With that trustworthy synthetic data, companies can then train models that can be safely and reliably used in the real world.
Synthetic data use cases
Synthetic data has various potential applications across almost all sectors. Its ability to mimic actual data makes it invaluable in business processes like development, testing, forecasting, and enhancing existing models. Some potential use cases include:
- Banks and financial services can use synthetic data to generate data essential in trading, customer service, and risk analysis. They can also create fake customer datasets to get around privacy concerns.
- Healthcare companies can analyse synthetic patient data without breaching privacy. This is crucial for sensitive medical information and could bring improved service and potential new knowledge.
- Structured synthetic data, by creating both categorical and numerical values, is a fantastic tool for software testing. Developers can quickly spin up artificial data lakes to train or test features, ensuring realism and quality without worrying about safeguarding real data. This could be particularly impactful in software development and cyber security.
- Some algorithms can create an almost infinite number of new, unique data points. One potential use is synthetic images teaching computer vision models about new or edge-case scenarios. Their unstructured nature is ideal for AI and ML models to learn new patterns. We’ve already seen Waymo use this technique to train their self-driving cars on more than five billion synthetic miles of driving data.
- Text is instrumental for tasks like data-driven sentiment analysis and building generative models. Using synthetic text, companies can make more intelligent models than ever.
- Above all, these synthetic datasets offer a simple alternative for AI and machine learning model development. If well-trained, they help build new models much more quickly, cheaply, and efficiently — all while ensuring safety and reliability.
How Nasstar and Colibri Digital can help
The potential advantages of synthetic data are game-changing. Any organisation using high-quality training sets with stringent data privacy can benefit from faster development, increased security, and new insights.
It’s important to remember that this powerful tool is not just for data scientists. Synthetic data can help many fields. From software development and business to highly regulated industries, almost everybody can use synthetic data to innovate efficiently and securely.
If you're looking to integrate synthetic data into your business operations, Colibri Digital (part of the Nasstar Group) has the expertise and infrastructure to help you leverage this technology effectively. Our services enable you to run your machine learning models with the enhanced capabilities and insights synthetic data can provide.
Embrace the future of data science and unlock new levels of efficiency and privacy in your business processes. Speak to a specialist to find out more.
FAQs
What is meant by synthetic data?
Synthetic data is new, non-real data created by advanced machine learning algorithms. It offers organisations a secure, cost-effective alternative to real-world data, bringing many potential business use cases. This artificial data, generated to replicate real-world data characteristics, harnesses generative AI techniques for authenticity and relevance. However, care must be taken to build synthetic data from quality sources to avoid bias and overfitting.
What is synthetic data vs real data?
Synthetic data, generated through machine learning algorithms, offers an alternative to collecting real-world data. In business, it might be used to train systems where new data is hard to come by, like medical information or banking transactions. But, unlike actual data, which often has limitations in size and scope, synthetic data is more accessible, flexible and scalable. It accurately reflects a broader range of values and behaviours, simplifying management and analysis.
What is synthetic vs dummy data?
Synthetic data is made by AI algorithms to mirror real data intricacies. Dummy data, on the other hand, is merely randomised, meaningless information used as a placeholder or for basic testing. A business could gain no usable real-world insights from dummy data. As the two are fundamentally different in complexity and application, distinguishing AI-generated synthetic data from mock or dummy data is crucial.